I recently had the opportunity to visit some of our financial services customers and leading partners to discuss the latest challenges and trends in their domains. During these meetings and discussions, we explored the transformative potential of scalable, high-speed software-defined storage (SDS) in modernizing datacenters to enhance real-time analytics and AI pipelines. The conversations underscored the crucial role of advanced storage technologies in enabling data-driven decision-making and operational efficiency. I would like to share with you some of my findings and observations.
Through these engagements, it became evident that the financial sector is rapidly evolving, and staying ahead requires leveraging cutting-edge storage solutions to handle vast amounts of data efficiently and effectively.
The Need for High-Performance Software-Defined Storage in Financial Services
Financial services increasingly rely on real-time data processing to make critical decisions, from executing split-second trades to assessing lending risks and detecting fraud before transactions are finalized. The rise of AI-driven applications presents a significant opportunity to extract valuable insights from these data streams. However, realizing this potential requires an infrastructure built on software-defined storage that can efficiently scale to accommodate the ever-increasing volume of data.
Benefits of Storage Disaggregation through Software-Defined Storage
When building a high-scale AI environment a significant optimization of storage & power consumption can be achieved through software-defined storage and storage disaggregation, akin to systems like NVIDIA’s SuperPOD. By leveraging SDS, financial institutions can:
- Reduce the need for dedicated SSDs per GPU: This minimizes stranded capacity per server and lowers the overall power consumption of the entire system.
- Increase system reliability: Software-defined storage creates an enterprise-grade, high-availability shared persistence layer, enabling faster recovery in case of GPU or SSD failure and improving the system’s mean time between failures (MTBF).
- Enhance resource utilization: Shared storage layers act as large caching systems accessible by multiple GPUs for tasks such as checkpointing, parameter sharing, and reload and recovery in the event of GPU failure.
NVMe/TCP: The Backbone of Modern Storage Solutions
NVMe over TCP (NVMe/TCP) has become a cornerstone technology for building scalable, high-performance infrastructure using a software-defined storage (SDS) storage model. This protocol enables direct access to NVMe storage devices over TCP/IP networks, bypassing traditional storage controllers and providing several critical benefits:
- High Performance: As opposed to “good old” iSCSI, NVMe/TCP’s parallelism ensures non-blocking connectivity, delivering consistent low latency and high throughput—essential for real-time analytics and AI operations.
- Scalability: Software-defined storage on NVMe/TCP can seamlessly scale to meet the growing data demands of financial services.
- Flexibility: This storage solution can be deployed both on-premises and in the cloud, offering financial institutions the flexibility they need to stay competitive.
Real-world Applications of Software-Defined Storage
Financial services companies are increasingly adopting software-defined storage built on NVMe/TCP to enhance their real-time analytics and AI capabilities. For instance:
- Risk Management Systems: A large investment bank uses SDS with NVMe/TCP to power its real-time risk management system, analyzing streaming data from market feeds and social media to identify potential risks
- AI-powered Customer Service: Another financial services company utilizes NVMe/TCP to boost the performance of its AI-driven customer service chatbot, improving response times and customer satisfaction
The Role of Retrieval-Augmented Generation (RAG)
RAG is becoming a foundational component in AI systems, especially for tasks requiring high accuracy and access to proprietary organizational knowledge. These systems need fast, scalable storage solutions to manage huge amounts of data, such as documents, images, audio recordings, and videos. Traditionally, these implementations relied heavily on in-memory databases. However, as data volumes grow, a fast storage layer optimized for vector databases becomes crucial. NVMe/TCP’s high performance and scalability make it an ideal choice for supporting the most scalable vector databases and real-time data integration within a software-defined storage framework.
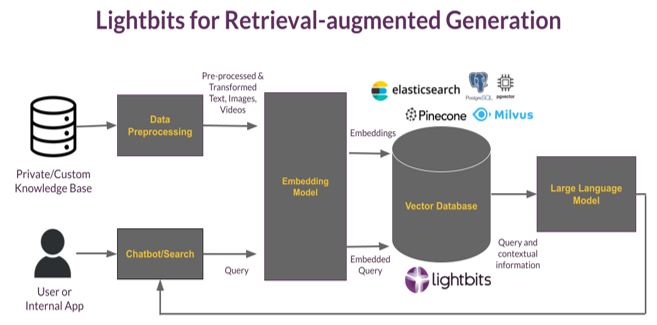
Lightbits high-performance, low latency software-defined block storage accelerates vector databases in LLM with RAG workflows.
Future Directions for Software-Defined Storage in Financial Services
The ongoing trend towards using NVMe/TCP in disaggregated storage solutions is set to revolutionize the infrastructure for real-time analytics and AI in financial services. By providing a robust, flexible, and scalable storage backbone, software-defined storage architected for NVMe/TCP empowers financial institutions to fully harness the potential of AI, improving decision-making processes, operational efficiency, and customer experiences.
Conclusion
Software-defined storage built on NVMe/TCP offers financial services companies the high performance, scalability, and flexibility necessary to support real-time analytics and AI pipelines. By reducing costs, power-consumption and enhancing agility, this advanced storage technology helps financial institutions maintain a competitive edge. As AI continues to evolve, embracing a software-defined, NVMe/TCP model for storage disaggregation will be critical for achieving higher accuracy and efficiency in data-driven decision-making. This approach not only addresses current data challenges but also lays a solid foundation for future innovations & scalability in financial services.
Additional resources: